With more traffic in Arctic waters, the risk of colliding with an iceberg can increase. If an accident occurs in these areas, darkness, cold and long distances will make rescue work more challenging.
In a project with Norwegian Coastal Administration (NCA) KSAT has used machine learning to discriminate between icebergs and ships. The aim of this project was to establish an automatic detection and delivery chain for reporting significant radar signatures that cannot be correlated with self-reporting from vessels, such as AIS.
The enormous area makes it challenging for the rescue service to operate everywhere with sufficient resources. Satellite-based monitoring is an effective contribution to building situational awareness and improving the decision-making basis, thus considered the most cost-effective way to monitor large areas.
That’s why preventive measures, such as the Norwegian Coastal Administration' s 24/7 operated maritime traffic centers that can monitor and control ship traffic, is a good idea. If the centers receive information of possible icebergs in their responsible area, they can warn the ships.
– The Norwegian Coastal Administration needs to know which of the objects detected are icebergs, so that the decision basis is as good as possible to be able to notify the ships, Karstein Ringjord says, who leads the R&D initiative from the KSAT side.
KSAT developed algorithms
When ships broadcast AIS (Automatic Identification System), KSAT can filter out these identified objects detected in the satellite image, and is then left with what are called dark detections. Still, there are still many radar detections that are not icebergs. For instance, not all vessels are obliged to use AIS or not using it due to other reasons, so you need to know if the radar is detecting a ship or an iceberg, or perhaps other objects, such as fishing gear or garbage floating around.
KSAT has therefore developed algorithms that can recognize different radar signatures. These algorithms use deep neural networks, meaning machine learning models that are taught using historical data.
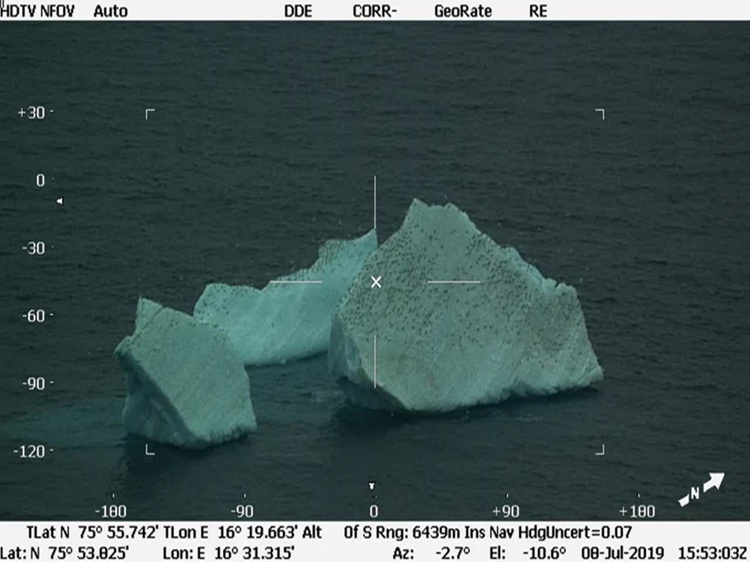
There are many examples that machine-learned models can perform tasks faster and more precisely than experts. In order to train a computer model to perform at the level of a human expert, one of the success criteria is that the training data is of high quality.
– To know that the quality is good, we wanted to verify the output, so that you know that the objects in the image are actually icebergs, Ringjord explains.
KSAT also added drift calculations for icebergs to our detections, which were developed by StormGeo. The idea behind this is that repeated detections that follow a calculated drift path for icebergs, with a higher probability can be assumed to be icebergs.
Crucial to detect ice
The Norwegian Coastal Administration (NCA) keeps a close watch on shipping activities in waters under Norwegian Exclusive Economic Zone (EEZ). This summer there will still not be a lot of cruise traffic, however there will be more expedition- and research ship traffic in the Arctic.
– All activity makes changes in the ice more critical to spot. This project shows that it’s possible to use machine learning for this, Ståle Sveinungsen, Head of Department for Ocean and Coastal Vessel Monitoring in NCA says.
NCA has responsibility for vast ocean areas and is constantly looking to improving the systems and automate the services in order to increase security at sea and provide maritime emergency response. They are sending out warnings of drifting icebergs and other threats to ships supporting safe navigation in the North.
– We have cruise ships sailing in the southern hemisphere the first month, then moving to polar areas the next. It’s important to give them warnings of changes in the sea well ahead of time, he explains.
2108 detections delivered
A total of 2108 detections were delivered from KSAT to NCA. By demonstrating an accuracy of well over 90 per cent from the satellite mode EW on Sentinel-1, which has a resolution of 25 meters, KSAT is optimistic when we expand the data models to other satellite modes with higher resolution. The project is considered a success, and KSAT and the Coastal Administration is aiming for a follow-up project.
Learn more: KSAT has set up a dedicated team to unlock the possibilities of Machine Learning.