Illegal, unregulated, and unreported (IUU) fishing causes an estimated annual economic loss of USD 26–USD 50 billion due to illicit trading of unreported catches of 8 to 14 million metric tons.*
Other nefarious maritime activities include illegal trans-shipment to circumvent international sanctions and various encroachments of state sovereignty. These actors typically disable or doctor the mandatory Automatic Identification System (AIS) messages. Therefore, Earth Observation imagery is essential to combating maritime crime, with KSAT playing a leading role historically and in the future. Deep Learning (DL) has enabled new higher levels of image analysis automation for whoever has data of sufficient quality and quantity.
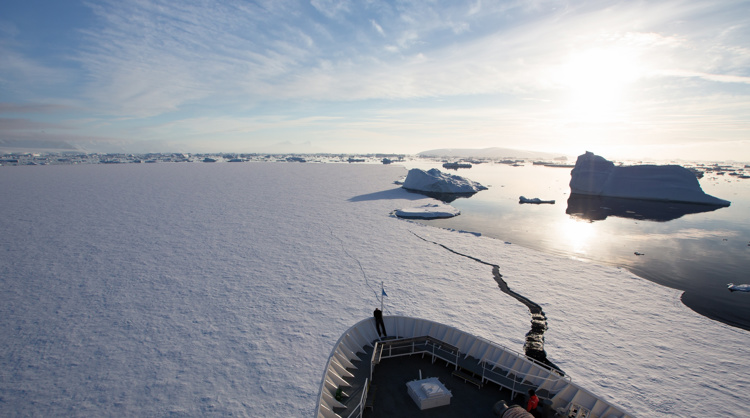
When it comes to maritime monitoring using Synthetic Aperture Radar (SAR) imagery, KSAT has the world leading data archives, a key prerequisite for a good DL algorithm. KSAT has invested significant time and effort into creating and refining these archives into a formidable data warehouse, which we believe will be pivotal in unlocking the potential of machine learning (ML) at KSAT. Our broad approach to DL automation includes all the SAR satellites in our arsenal, including high resolution modes. Our ambition is to tell our clients ever more about what’s in their areas of interest, and DL is the key to eliciting more information about dark targets (vessels that for some reason do not emit AIS messages) including vessel type and size. But we don’t limit ourselves to SAR vessel detection: We currently have pre-production DL prototypes for oil spill detection and both low- and high-resolution optical vessel detection.
In 2020 our ML effort blossomed. In the summer months, our pilot model for detecting objects at sea and discriminating icebergs from vessels helped The Norwegian Coastal Administration monitor the seas around Svalbard, where the combination of expeditionary cruises with thousands of passengers, icebergs, and limited rescue resources has been identified as high risk. That delivery embodied the frame of mind that “if we can do it here, we can do it anywhere”, since monitoring is a notoriously difficult task within SAR maritime surveillance. Hence, we are optimistic about our prospects of automating large parts of our services, both delivering human out-of-the-loop services and empowering our experts to deliver KSAT’s manual analyses with increased speed and efficiency.
Coupled with the availability of free data from the Copernicus Sentinel mission, we envision a near term future with distinct service levels, from copious free data with fully automated DL analysis, to high resolution imagery assessed by experts. And of course, continuing to push the limits when it comes to timeliness. Implementing ML/DL models in a way which satisfies KSAT’s commitment to quality and reliability means that “just” training a good model and putting it into production is insufficient. A full MLOps approach is needed, integrating data and ML models into the continuous integration and delivery cycles of the DevOps workflow. At the time of writing, we have a double digit number of ML models being phased into our production chain, relying on our top Earth Observation experts for assessment and MLOps best practices for reliability, stability, and scalability.
* https://advances.sciencemag.org/content/6/9/eaaz3801